Machine Learning in Marketing: Role, Tips & Examples
Of the many subsets of AI, perhaps machine learning is most common in marketing: it ensures data-driven decisions and helps optimize campaigns.
This technology can be applied in marketing to a whole host of aspects of the customer journey-from personalized recommendations to predictive analytics.
In this blog, we will talk about the increased utilization of machine learning in marketing. From its role to its benefits, you will get to know about several aspects involved in this blend.
What is Machine Learning?
Machine learning is an innovative technology that is revolutionizing business operations. In this way, machine learning, as a part of artificial intelligence, gives computers the potential to learn from data and perform better over time.
While traditional programs have to be explicitly instructed, machine learning algorithms can pick out patterns, make predictions, and sometimes even decisions without explicit programming. The capability of learning and adaptation makes it a very powerful tool for businesses seeking to get an edge over their competitors.
Machine learning has been applied to marketing in the performance of automation, personalization of customer experience, and optimization of SEO campaigns. Machine learning enables this business to make decisions with data-driven inputs, improve efficiency, and, as a result, bring in better results.
Impact of Machine Learning in Marketing
With the transformations that machine learning can offer, it will be increasingly common to see its integration into marketing strategies in quite a substantial number of businesses. The growing adoption of AI and ML in marketing reflects a turn towards more data-driven, personalized ways, and efficient ways.
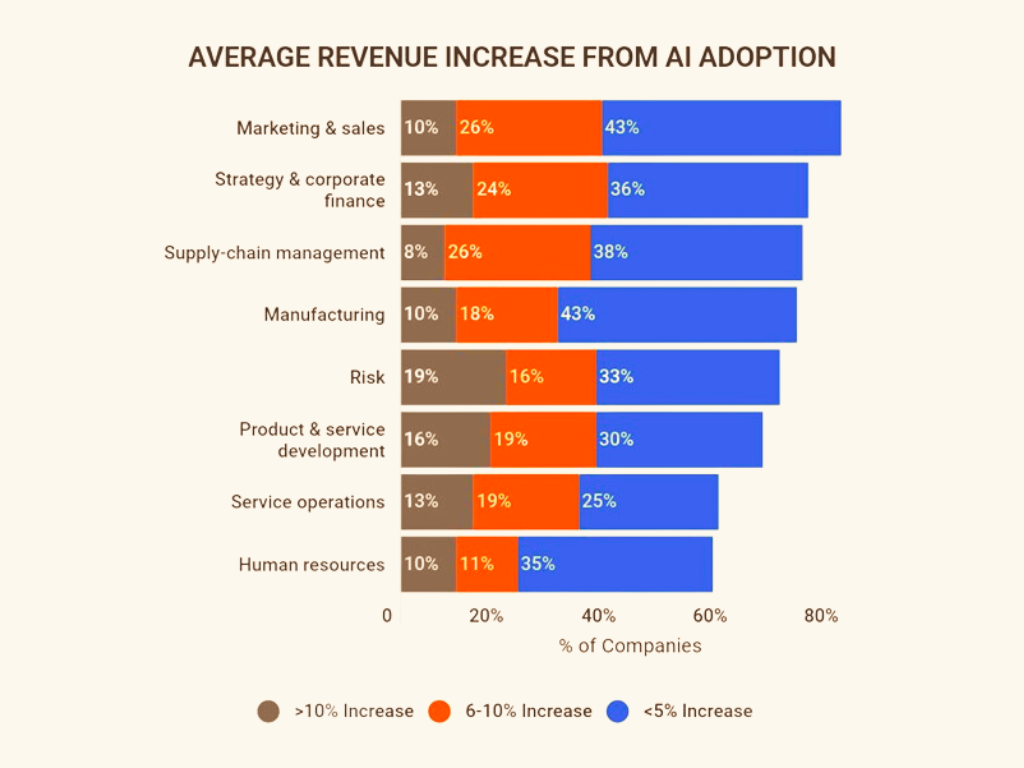
With the evolution the continuance of these technologies, we shall only see more inventive applications and transformative effects on the landscape of marketing.
- 60% of the marketers have a well-defined strategy related to AI.
This shows there is increasing awareness for the potential beneficence coming from AI and ML in marketing – and how to proactively merge these technologies into business operations.
- 78% of marketers say their customer engagements are data-driven.
This shows the direction of making analytic-based, data-driven marketing decisions—where a business formulates its developments on analytics to understand customer behavior and preferences.
- 51% of marketers believe AI to be very essential for marketing success.
This figure shows the increasing importance that developers attach to AI as an essential tool for meeting marketing objectives and remaining competitive.
- 74% will intelligently automate over 25% of their tasks
That would support the general trend of automation: through AI, marketers are simplifying and smoothing processes.
Hence, machine learning’s early adopters can give businesses the significant edge needed to maintain the benefits of these technologies in support of businesses.
How is Machine Learning Used in Marketing?
Machine learning has brought a revolution to the marketing landscape in terms of enlightenment in insights and automation of processes. With machine learning, marketers can reach deeper insights into customers, optimize campaigns, and serve up more personalized and effective experiences.
Following are some of the key ways it finds its application:
1. Content Optimization
With machine learning algorithms, an immense amount of data is analyzed on what topics, formats, and styles will work best with your audience.
Accordingly, machine learning might make recommendations for relevant content to individual users based on user behavior and preference, driving engagement and ultimately conversion.
2. Human-Machine Collaboration
While humans are still essential for creating high-quality content, machine learning can assist in generating ideas, optimizing headlines, and suggesting relevant keywords. Machine learning can automatically curate content based on user preferences, saving time and effort.
3- Human-Machine Collaboration
While this is a task still laboriously requiring human input-create high-quality content-machine learning can help with everything from idea creation, and headline optimization to suggesting relevant keywords. Machine learning can automatically curate content based on user preferences, saving time and effort.
4- Customer Journey Analysis
With machine learning algorithms, the user’s behavior on your website can be tracked from the very visit to the conversion. This helps identify different bottlenecks in the customer journey and optimize a website accordingly to boost conversions.
Tips to Utilize Machine Learning to Boost Marketing
Machine learning provides a powerful toolkit that marketers use for the optimization of their campaigns and the delivery of personalized experiences to improve results.
Here are deep insights into how to get the best out of machine learning:
1- Define Clear Objective
Clearly define your objective of marketing-for example, is it to increase sales, enhance customer satisfaction, or improve brand awareness? Make sure your machine learning efforts align with the ultimate business strategy and objectives of the work.
2- Data Collection and Preparation of High-Quality Data
Pull data from multiple sources such as your website, CRM system, social media, and your automation tool. Data cleaning and preprocessing example, fixing errors and inconsistencies, filling in any missing values, and creating features or variables that will be used for the machine learning algorithms.
3- Select Appropriate Machine Learning Models
Choose algorithms for classification, regression, or clustering that best fit your objective. The best algorithm should be determined by trying a few of them and measuring the results against one another. This makes sure that the best-fitting algorithm is selected to work with your data and objectives.
4- Train and Evaluate Your Model
Make sure the training dataset you have prepared is similar to those in the real world that your model will be exposed to. The predictions can also be biased if the data is biased. Split the data into training and testing sets. You train the model with your training set, but test its performance on data it has never seen with the testing set.
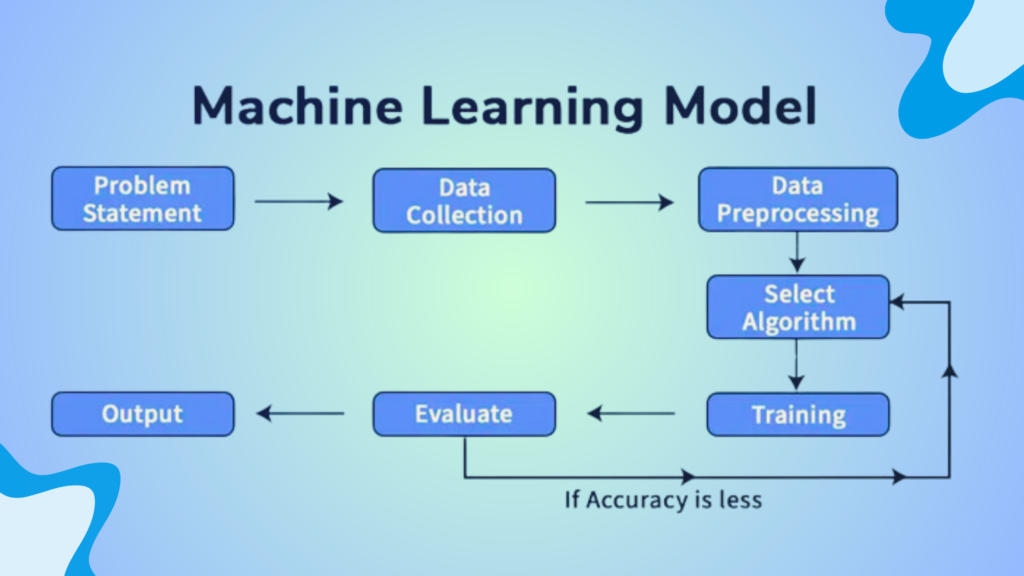
Use cross-validation techniques to evaluate model generalization ability, avoiding overfitting. Overfitting means when a model does not generalize well to new, unseen data, after having done well on the training data.
5. Implement and Monitor
Seamlessly integrate the trained machine learning model into existing marketing workflows and systems. Continuously monitor the performance of the model to identify any issues or areas of improvement. The model will be regularly retrained with new data to stay accurate and up-to-date.
6. Leverage Cloud-Based Platforms
Cloud-based platforms boast scalable infrastructure where resources can be adjusted according to requirements. That too, without a high initial investment, since most cloud platforms provide pre-built machine learning tools and libraries that quicken the pace of development and deployment.
Utilize managed services for infrastructure management and maintenance by-scale resources to allow your teams to devote more time to model development and optimization.
7. Ethical Considerations
Make sure to follow privacy data protection regulations like GDPR and CCPA, protecting customers’ private information. Be aware of how biased datasets and algorithms can create prejudice. Ensure that the models you create use techniques like bias detection and correction.
Provide people with enough transparency so that they understand how your machine learning models work and how decisions are made. This will help establish trust with your customers and other stakeholders.
Machine Learning Applications in Marketing
The modern marketer finds machine learning an indispensable tool: it allows them to base their decisions on data and optimize their campaigns. In turn, marketers can procure gleaned insights on customers so they can do their best when creating campaigns that will lead to the best business outcomes.
Here are some key applications of machine learning in marketing:
1. Customer Segmentation
With machine learning algorithms, customer segments based on psychographics, purchase behavior, and even sentiment analysis can get very granular beyond simple demographics.
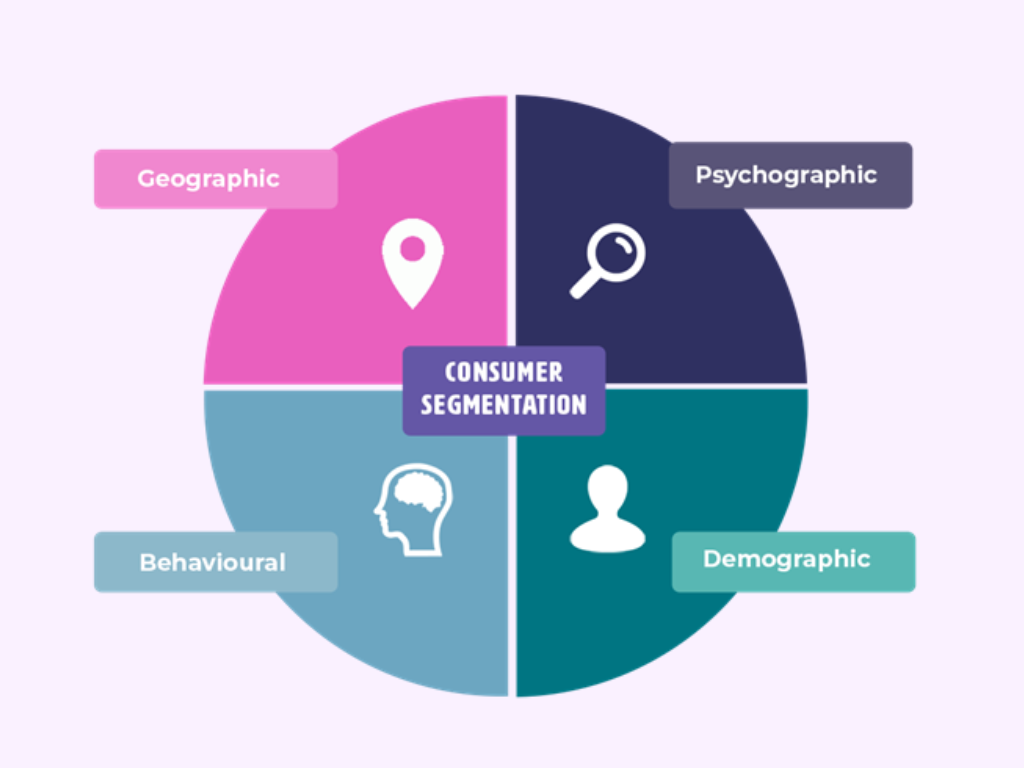
Tailor marketing messages and offers to specific segments so that customers only receive content relevant and of value to them. You also use machine learning to optimize your marketing campaigns by testing different messages, offers, and channels for each segment.
2. Recommendation Systems
Suggest similar products or services based on the preferences of other customers with similar tastes. Recommend items based on the content or attributes of products or services that a customer previously showed interest in. Combine collaborative filtering with content-based recommendations to make suggestions both more accurate and relevant.
3. Optimizing Marketing Campaigns
It involves the use of machine learning in making data-driven decisions to optimize marketing campaigns. This helps marketers instead of simply assuming or intuitively making decisions may allow data-backed insights to drive them to:
- Target the Right Audience: The most workable channels and demographics they could use to reach their target audience.
- Personalization of Messages: This refers to sending messages that can best identify with each of their customers.
- Campaign Effectiveness: By monitoring KPIs, your business can judge the effectiveness of running marketing campaigns for proper adjustment where necessary.
- Maximum ROI: The effective use of finite resources to market better and achieve maximum ROI.
4. Customer Churn Prediction
Examples of early warning signals include decreasing activity, rising support queries, and changed purchasing habits. This means designing focused campaigns that appeal to the needs of at-risk customers and incentivize them to stay on board by offering either a discount, loyalty program, or more personalized service.
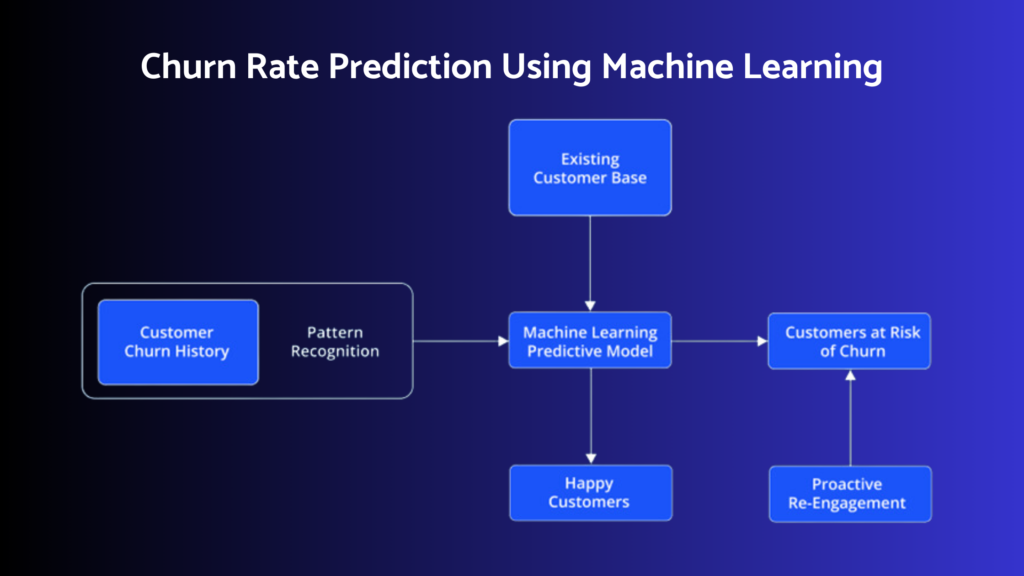
5. Lifetime Value Forecasting
Estimate the CLV according to purchase frequency, average order value, and customer lifetime. Pinpoint which high-value and high-margin customers would be worth a lot in terms of revenue for your business. Allocate the right marketing resources since you would understand the relative value of different segments.
6- Managing and Analyzing Large Datasets
Today’s tsunami of data, however, may get analyzed in less time than the human analyst can achieve. In contrast, machine learning algorithms are designed to process large volumes of data efficiently and transform this into meaningful insights. This would otherwise remain hard or impossible to find by humans.
Examples of Machine Learning in Marketing
Brands today are using machine learning models to amplify their marketing activities resulting in a huge spike in their conversions. We are here to discuss the top brands that are reaping the huge benefits of using machine learning in marketing.
1- Spotify
Spotify, the leading music streaming company, successfully deploys machine learning in predicting customer churn and proactively applies customer retention strategies. Spotify identifies the customers likely to leave them through demographic data analysis, past user behavior, and other factors.
This predictive capability allows Spotify to adopt pre-emptive measures for retaining customers, such as offering lower subscription rates or personalized recommendations.
With Spotify, one can significantly improve customer loyalty by even anticipating customer churn and taking up the issues much before they become issues, thereby driving revenue growth and improving the bottom line.
2- Netflix
The integrated AI system for Netflix can predict which movies or shows a user would rather see.
For example, if one begins watching a movie but then loses interest, the Netflix algorithm may then offer alternatives that align better with the respective user’s tastes and preferences. This personalized approach has been critical in minimizing churn and maximizing customer retention.
According to The Motley Fool, Netflix estimates that its recommendation engine saves the company a whopping $1 billion every year. This is a good example of how AI is improving business bottom lines.
Netflix hugely succeeded with personalized recommendations via machine learning integrated with its content recommendation platforms.
3- Twitter
Twitter is more than just a social network; it’s also a powerful consumer insights engine. With machine learning, there are various features Twitter has set in place to make the experience far more user-friendly, and even relevant for today’s time.
Automation in Twitter algorithms selects the most visually interesting part of the image to show for a better visual experience by the user. Twitter deploys machine learning algorithms to help identify and filter out hateful content, turning it into a much safer and more inclusive online community.
The analysis of user behavior and preference can therefore lead Twitter to suggest other timelines and content, keeping users engaged in finding out more about this.
4- DoorDash
The most important means by which machine learning was used to help DoorDash cut into marketing spending is by using AI-drive algorithms. This strategy helped the company reduce marketing spending by 10-30% while not losing customers.
This is a clear indication of the strength of machine learning to solve one of the issues that have persisted in business entities across the world – how to utilize marketing budget to get optimal and enhanced returns.
Machine learning algorithms will go on to analyze the granular volumes of data to identify the best channels for marketing, audience targeting, and messaging strategies that work: thus, enabling resource allocation within companies. This way businesses can best utilize their resources for the best results.
5- Airbnb
Airbnb deploys predictive modeling to make highly personalized recommendations to its guests. Suitable accommodation for individual preferences and needs, according to user preferences, location factors, and previous stays, is what Airbnb is capable of recommending.
Recognizing the challenges hosts face in determining optimal pricing, Airbnb uses AI to dynamically adjust pricing not only by supply and demand but also by listing specific details. This intelligent pricing system will ensure that hosts can earn the most while being able to attract guests.
It uses AI in screening guests who come onboard, using data from third-party sources to ensure this platform remains safe and secure, keeping off fraudulent activities that may affect even the hosts or guests themselves.
The single largest use of machine learning at Airbnb to date has been one of the major reasons for its phenomenal success, right up to an extremely high valuation IPO, higher than Marriott and Hilton put together. With AI, Airbnb has proved beyond doubt that it will continue to outperform the competition and offer better value to its consumers.
Key Takeaways
Machine learning has become a powerful tool that can help marketers optimize their campaigns, make experiences more personalized, and drive better results. This means companies can get important insights, automatize tasks, and make data-driven decisions in their work by applying machine learning.
This, therefore, calls for more innovative applications of machine learning in enhancing marketing soon, with further improvements in technology. Through this power tool, businesses can thus attain a competitive advantage besides ensuring long-term success.
eSearch Logix continuously updates and optimizes marketing and branding strategies with the advent of the latest technologies like machine learning. We are helping brands stay competitive through our business-oriented approach, merged with innovative platforms.
So, if you are experiencing the heat of increased challenges in the current landscape, partnering with us will be the right choice for your brand.